Introduction: AI Implementation in Business
In a recent study by Dataiku, 74% of 500 CEOs worldwide confessed they could lose their jobs within two years if they don’t deliver measurable AI-driven gains. That’s not speculation; it’s their own words. (Dataiku, 2025)
Concerns about AI implementation in business aren’t limited to employees anymore. Leadership teams are now facing their own version of uncertainty. Half of all CEOs surveyed believe AI can replace 3–4 executive team members for the purpose of Strategic Planning (Dataiku, 2025). This means that as AI progresses, it is defining leadership itself. And the pressure is mounting. CEOs are being asked, both directly and indirectly, to prove AI implementation in business can deliver real business value, in the short and medium term. Below is a snapshot of how many executive roles CEOs believe AI can replace, broken down by region, underscoring the growing concern and sense of urgency around AI adoption.

Fortunately, the upside is real. Microsoft found that AI investments yield an average return of 3.5x. Top performers are seeing returns up to 8x. (Microsoft, 2024)
This raises a clear question: What is the CEO’s role in implementing AI in their company?
More leaders are realizing it can’t be delegated. Approving budgets, reviewing adoption metrics, or benchmarking peers may offer visibility — but they don’t create momentum. Driving AI implementation in business requires top-down involvement. It means setting direction, removing structural blockers, and holding the organisation accountable for outcomes. That shift is already underway, and it’s becoming a defining test of leadership.
The Role of the CEO in the Age of AI
Alexander Sukharevsky, senior partner and global coleader of QuantumBlack, AI by McKinsey, explains that
“Effective AI implementation in business starts with a fully committed C-suite and, ideally, an engaged board. Many companies’ instinct is to delegate implementation to the IT or digital department, but over and over again, this turns out to be a recipe for failure.” (Singla et al., 2025)
The same report by McKinsey also reveals that
“CEO’s oversight of AI governance—that is, the policies, processes, and technology necessary to develop and deploy AI systems responsibly—is one element most correlated with higher self-reported bottom-line impact from an organization’s gen AI use.”
This is why it’s extremely important for CEOs to be involved in AI initiatives. Their responsibility is not merely limited to short-term outcomes but also involves setting the direction for tomorrow. That’s where futures thinking becomes essential (Future Thinking: What It Is and Why It Is Important at Work, 2024).
It’s about asking:
- How will AI change my business?
- Where are we in the adoption curve?
- How fast are our competitors moving?
- Are we already behind?

One of the clearest examples of this mindset comes from Moderna. In a 2024 case study published by OpenAI, the pharmaceutical company deployed ChatGPT Enterprise across the business — not as a tech project, but as a core capability. Their goal: 100% organizational proficiency within six months.
Legal, commercial, R&D, and clinical ops were empowered to create AI tools that improved daily work. In just two months, employees built over 750 internal GPTs. Leadership didn’t just endorse this — the CEO and executive committee actively drove the rollout.
The tech mattered, but what set them apart was posture. Moderna didn’t wait for AI to become mainstream. They recognized the shift and acted early — with urgency, not hesitation. (Open AI , 2023)
This is what futures thinking looks like in practice: exploring how the company performs in different future scenarios, then preparing to win in the one most likely to emerge.
Consider Your Industry
AI implementation in business is accelerating, though not at the same pace across industries. Sectors like marketing and sales have already moved into active deployment, with 67% of companies actively using AI (See Image below). In these spaces, AI is no longer experimental; it is built into daily operations, and expectations around performance and speed have shifted accordingly.
In contrast, industries such as supply chain, legal services, and parts of manufacturing are still in earlier phases of adoption, with only 12% of companies currently using AI. For organizations in these slower-moving fields, this lag presents a clear opportunity. Moving early can offer not just internal efficiencies but a significant competitive edge. In particular, implementation of ai in healthcare is another area that can see large gains for early movers.
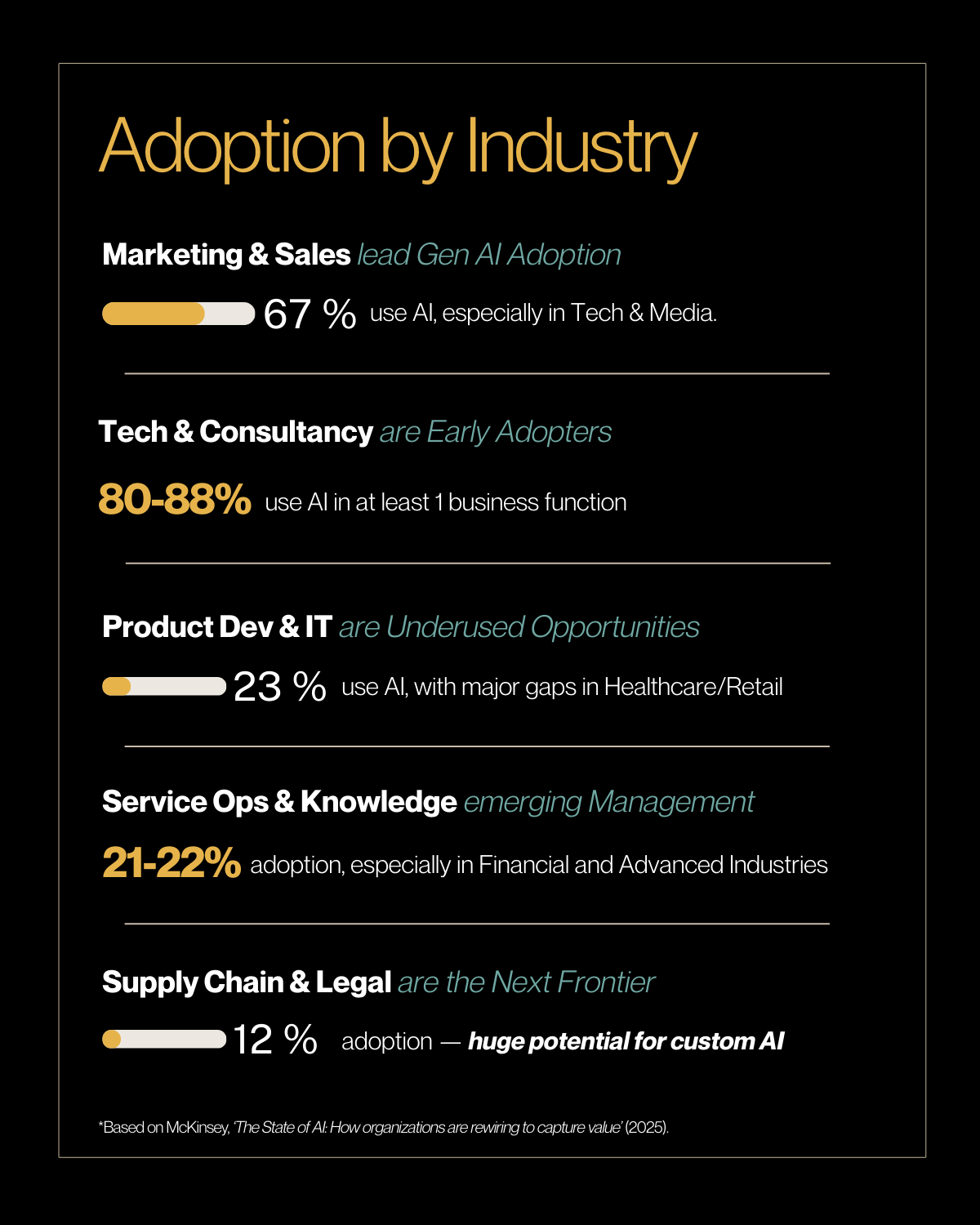
Where AI is already embedded, adoption is expected. Where it is still new, it can become a source of advantage. Knowing where your industry stands helps clarify the urgency—and the opportunity.
The goal is not to panic. It’s to prepare. Strategic leadership isn’t about reacting to trends. It’s about creating the environment where the company can move deliberately and decisively.
When it comes to successful AI implementation in business, it’s vital to explore a wide range of options—whether it’s generative AI implementation or more traditional approaches. Our AI Quickstarter program helps you pinpoint the right strategies and deliver fast, impactful wins in just four weeks.
How to Implement AI in Your Company
Too many AI rollouts start with tech and end in disappointment. The companies that succeed treat AI as a business decision, not a software decision. That means starting with what matters most: your people, your systems, your economics, and your ability to execute.
Start With Business Problems, not Technology Solutions
AI creates value when it’s linked to friction in the business, not when it’s deployed for its own sake. Most failed implementations can be traced to solutions in search of a problem.
A more strategic approach starts by identifying core constraints: where margins are eroding, decision-making is too slow, or human capacity is spent on low-leverage tasks. These bottlenecks usually present as cost inefficiencies, customer churn, or flat growth despite rising operational complexity.
AI becomes useful once you isolate the source of the constraint. For example, if B2B sales conversion is lagging, the issue may not be sales talent, but fragmented customer data across functions. In that case, deploying a generative ai implementation for proposal writing isn’t the solution. It’s the endpoint of a broader redesign that includes pipeline intelligence, lead scoring, and shared CRM infrastructure.
Strategic framing: Don’t ask,
"What can AI do for us?"
Ask,
"Where are we failing to scale, and what role could AI implementation in business play in unblocking that?"
Assess Systems and Data Readiness
Before moving forward with any AI initiative, it’s worth pausing to ask a more basic question: are we truly ready?
This goes beyond having a pilot program in place or testing a tool on a small team. Real readiness means the organization is set up to make use of AI consistently, at scale, and across business functions. That begins with the fundamentals: your data and your systems.
If your data is inconsistent, messy, or incomplete, then any effort to use AI will reflect those weaknesses. Andrew Ng, a leading figure in the AI space, once said:
“Data is food for AI. If you have bad food, no matter how good your chef is, you're going to get a bad meal.”
In short, poor input leads to poor output. It doesn’t matter how sophisticated the model is if the underlying data can’t support its decisions.
Leaders need to evaluate three things to understand their organization’s level of preparedness:
- Data Readiness: Is the data clean? Is it labeled in a way that machines can learn from it? And is it stored in a place where it can be traced, audited, and updated?
- System Readiness: Are systems integrated in a way that makes relevant data visible and usable? Can insights be generated quickly, or does everything still run through disconnected platforms and outdated processes?
- Organisational Readiness: Do teams understand what AI is meant to do? Are there processes in place to actually use what the tools generate? Do people know who is responsible for what when AI gets involved?
AI will not fix misaligned teams or chaotic workflows. It will make those issues more obvious. If the groundwork isn’t solid, early AI experiments will likely stay small, isolated, and unable to scale.
In cases like these, having an external perspective can make all the difference. That’s why the AI Quickstarter includes a complete technical audit—helping you assess data quality, system readiness, and integration gaps before you scale. Over the course of four weeks, we work directly with your leadership team to map high-impact opportunities, evaluate your infrastructure, and deliver a tailored AI roadmap. This ensures you're not just experimenting with AI—you’re building on a solid foundation with clear ROI and long-term value in mind.
Build a Financial Case With Real Numbers
AI should be evaluated with the same discipline as any capital investment. That means putting aside the buzz and focusing on fundamentals: cash flow, risk, and long-term return. If you wouldn't approve a new product line without a proper investment case, AI implementation in business deserves the same level of scrutiny.
Start with a baseline financial model. Think about:
- Assumptions about performance improvement: Document these clearly. What specific part of the process is being improved, and by how much? Is it a reduction in manual effort? A decrease in rework? Faster delivery times? These assumptions must tie directly to how the work gets done.
- Total cost of enablement: This means everything required to get the AI solution live and working. That includes data preparation, integration with internal systems, training time, and any external support. Be honest about internal capacity. If people need to shift focus to support the rollout, that has a cost — usually in delayed work elsewhere.
- Opportunity cost: Every resource committed to AI is a resource not used elsewhere. If your data science team is building this model, what else could they have done instead? If your operations lead is tied up on integration, which other projects are on hold? The trade-offs matter.
- Risk-adjusted net present value: Factor in the time it takes to implement, how quickly teams will adopt the new process, and where resistance might slow things down.
Also, keep in mind sunk cost. Just because you’ve already paid for a pilot or invested in tooling doesn’t mean you need to double down. If the numbers don’t work, or if organizational readiness isn’t where it needs to be, walking away is not failure. Sunk cost shouldn’t drive strategy.
The best financial models also account for second-order effects. These are the less obvious, but often more powerful, benefits. For example, an AI tool that improves inventory accuracy may look modest on paper. But if it leads to faster lead times, more flexible pricing, or better vendor negotiations, the return on investment grows exponentially. That’s not fluff, it’s real operational leverage.
This is where many AI business cases fall apart. Not because the value isn’t there, but because no one takes the time to model it correctly. The analysis is either too shallow or too focused on short-term gains.
AI can absolutely drive financial return. But it only becomes a smart investment when it’s evaluated with the same care, realism, and rigor as any other strategic bet.
Prepare the Organization
AI does not generate value on its own. Its usefulness depends on whether people understand how to work with it — and whether their roles are set up to support that shift.
This is where many AI rollouts begin to stall. Tools are introduced, but workflows stay the same. The result is visible activity without meaningful impact.
Build capabilities around how work actually happens
Training should reflect the specific responsibilities of each role. Different parts of the organization engage with AI in different ways, and the skills required should match that reality.
- Executives need to understand how AI informs business priorities. They should be fluent in how it affects portfolio decisions, strategic risk, and long-term governance.
- Managers benefit from direct, hands-on experience. They need to understand prompt-based assistants or generative ai implementation interfaces so they can reduce complexity in team operations and drive ai for operational efficiency.
- Staff need to develop the skill of working with AI output. That includes interpreting suggestions, validating results, identifying errors, and recommending improvements. These are applied, judgment-based skills that improve over time with real use.
Measure the Right Outcomes
Tracking AI usage is easy. Measuring impact is harder — and far more important.
Many teams report adoption metrics: logins, usage frequency, number of prompts. But unless those metrics link directly to business performance, they’re noise.
The right approach is to tie AI efforts to the specific business problem being addressed, and measure success through that lens. If the objective is to improve demand prediction, success isn’t measured in model output or dashboard activity. It’s measured in outcomes like forecast accuracy, inventory turnover, or stockout reduction — all of which tie back to working capital and margin.
This logic applies across use cases:
- If you’re automating customer support, track resolution time or ticket deflection rate.
- If you’re optimizing pricing, monitor conversion rates or average deal size.
- If you’re deploying AI for internal reporting, measure time-to-insight or decision latency.
- If you’re exploring implementation of ai in healthcare, measure patient outcomes or reduced wait times.
Be careful not to fall into the trap of optimizing proxy metrics. When teams focus on what’s easy to measure rather than what matters, effort gets misdirected. As Goodhart’s Law reminds us: when a measure becomes a target, it ceases to be a good measure.
Conclusion
The companies that succeed with AI implementation in business don’t just monitor usage — they manage impact. They define success the same way they would for any strategic initiative: by whether it moves the numbers that matter.
If you're ready to move from uncertainty to impact, the AI Quickstarter is a focused 4-week program designed for high-growth companies looking to implement AI with speed and clarity. It combines a full technical and strategic audit, opportunity mapping, ROI modeling, and leadership upskilling—all with hands-on expert support. You’ll walk away with a clear, actionable roadmap tailored to your business. No fluff, no guesswork—just measurable outcomes from the start